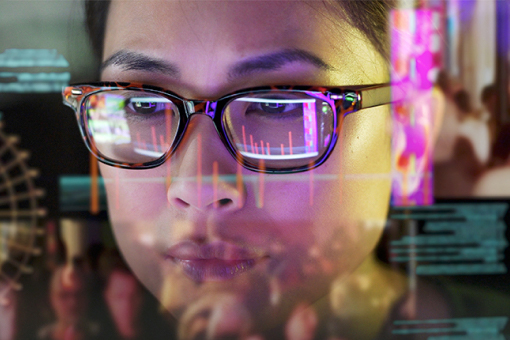
Stay on top of Current Trends
Stay up to date with the latest learning technology research, events and funding opportunities.
We will collect, use and protect your data according to our privacy policy.
Post by Niamh Walsh, Learnovate
Interest in artificial intelligence (AI) and machine learning (ML) has exploded, and investment is following swiftly and growing exponentially. With easy access to processing power, accessible ML tools, reliable connectivity, and mind-boggling expanses of data, companies are hungrily eyeing machine learning capacities to improve productivity, enhance customer engagement and ultimately boost the bottom line.
While R&D-hungry multinational tech companies gobble up machine-learning startups as acquihires or frantically upskill their in-house teams to keep up with the competition, there is a glaring gap in the adoption of machine learning in one vital area of today’s organisations: human resources (HR). Learning and development (L&D), located so often within the HR function, is similarly untouched by advances in ML techniques. As AI and ML transform businesses and disrupt sectors, why has this key function remained relatively immune?
The ingredients for machine learning are good, clean data (and lots of it) and at least one sensible, intelligent human to plan the process, pick the algorithm and test the results. Needless to say, you won’t get far without a clear understanding of the problem that you want to solve using ML. Most ML applications use ‘supervised’ learning where the algorithm is trained to recognise a desired pattern in existing data. The application’s performance can be tuned during this training phase until it produces results that are accurate enough to be applied in the real world.
Training machine learning applications is a little like training sniffer dogs to search for narcotics and explosives at ports and airports. When training a sniffer hound, the owner presents objects with the scent she wants the dog to detect. Once trained, the dog can detect a selection of scents in packages, luggage, vehicles and premises. In machine learning, you present training data to the algorithm so it can learn how to search through target data to find things that match the patterns it has learned to identify. Frequent applications of supervised learning include searching through historical data, where there is too much information for a human to handle, and using past outcomes to predict future trends.
With supervised machine learning, you train the machine learning application to search for a result. Like training a sniffer dog, you know at the start what you want your dog to find.
With its far less common cousin – unsupervised machine learning – the algorithm is unleashed to nose around the data in its own way to see if it happens upon secret treasure. Just like Monty the dog, who amazed his owner this year by digging up a cache of Bronze Age artefacts on his regular walk near his home in the Czech Republic, unsupervised machine learning algorithms can uncover unseen patterns or hidden relationships in the data that generate valuable knowledge for the company.
Pictured above: Bronze Age relics discovered by Monty the dog in the Hradec Králové region of the Czech Republic in 2018 via Smithsonian Magazine
Whether supervised or unsupervised, a human is also needed at the end of the process to interpret the results. In the training phase of supervised learning, it’s easy to evaluate the effectiveness of your chosen algorithm because you define a set of desired outputs in advance. Like Monty the dog’s owner, with unsupervised learning, the ‘treasure’ is dumped unceremoniously at your feet and the human needs to examine it to figure out if it’s actually treasure or just an old boot.
Look what I found! With unsupervised learning, the machine learning algorithm is let loose on the data to see what it might discover.
Learning management systems (LMS) and HR platforms generate extensive amounts of data that may provide rich pickings for machine learning algorithms. The oceans of data generated by learning platforms continue to grow with the use of more data tracking, analytics and the emergence of learning data standards such as xAPI. Already drowning in data, companies can’t cope with the increasing quantities of data their systems are generating. Like straw piled to the roof in the Brother’s Grimm tale ‘Rumpelstiltskin’, companies have no idea how to spin their data into gold with current technologies. Companies need the ‘magic’ of new technologies like machine learning to take the burden of overwhelming data out of their hands. Like other functions in the organisation, HR and L&D will inevitably turn to machine learning to deal with the large volumes of data that they can no longer manage efficiently or effectively using traditional data processing techniques.
Managers are overwhelmed by the oceans of data generated by applications throughout the organisation.
There is no doubt that ML has the capacity to provide critical insights to HR to inform organisational decision making, and help L&D more effectively support learners’ needs. Machine learning has already proven its worth in web search, email spam filtering, marketing personalisation, product recommendation, dynamic pricing, financial trading, fraud detection and chatbots. While the learning domain often lags behind other fields in technology adoption, leading companies in the corporate sector – reaping the benefits of machine learning in other contexts – are now beginning to apply ML in educational and performance contexts.
While there are multiple areas ML can create impact in these contexts, it’s currently applied most often to solve challenges in analytics and reporting, feedback and assessment, and personalisation and recommendation. Early adopters are already gaining key insights by applying ML algorithms to analyse patterns in employee and learning data, offer recommendations, make predictions, and deliver supportive interventions.
Machine learning may help HR to learn new tricks that provide critical insights that inform organisational decision making and support employee development.
Existing learning and performance platforms are already applying ML to provide enhanced analytics and there are key areas that demonstrate early impact. This includes improved forms of analytics and reporting of learning and performance data. The goal from the application of ML in this context is to gain more actionable insights that are based on past activity and likely future outcomes. Companies can use this information to develop more detailed and real-time reports on effectiveness and return on investment (RoI) of learning within an organisation. This helps learners, trainers and the organisation to better understand learning and how it functions in the organisation. An example is the ability to identify trends and take pre-emptive action, such as supporting learners at risk of not completing a course. Organisations can use this type of data insight to improve employee retention by identifying development needs and supporting employees proactively. Organisations are already starting to use the predictive benefits of ML for:
Machine learning can empower applications to provide more advanced feedback techniques – delivering automated, timely, relevant, individualised feedback to learners informed by real-time data processing by ML algorithms. An ML application can process aggregate learner data to predict the optimum occasions to provide feedback and identify the format feedback should take. ML techniques can allow feedback to take place when learners are in the flow of learning, when it may be most effective. ML techniques enable systems to deliver intelligent automated feedback, such as advice on skills gaps, directly to learners.
ML can be a driving force behind more efficient online assessment strategies. By processing large amounts of user data, applications can create more intuitive, intelligent tests and quizzes for learners. Some systems can automatically formulate appropriate test questions in reaction to learner activity. This can be delivered when needed to provide dynamic and effective assessment strategies within learning platforms.
Personalisation and recommendation of learning resources is a common current use of ML in learning platforms. In this context, ML interprets large volumes of data to match appropriate content to learners’ needs based on their, and other users’, previous activity. This allows more targeted planning and development using learning behaviours, performance indicators, and emerging patterns to personalise online training.
ML is already implemented in some of the large learning platforms to drive more intelligent content recommendation for learners. This helps users find more relevant content from the increasingly large volumes of content available, both in internal knowledge repositories and on the web. ML enables systems to offer personalised training paths and adaptable online training resources based on data about employees’ learning experiences and assessments.
Corporates, higher education institutions and schools are all focused on the early identification of learners who are at risk of disengaging and failing to complete their learning objectives. Learning analytics and machine learning techniques can identify trends in learner data that empower organisations to identify at-risk learners earlier and with greater accuracy.
Identification of at-risk learners improves the learner experience by providing necessary support earlier in the learner’s journey. As well as supporting at-risk learners, ML can help organisations identify high-performing learners or other learner groups. This type of insight can enhance the organisation’s learning strategy and increase learning effectiveness by tailoring learning to the needs of specific learner cohorts.
We are already familiar with chatbots and virtual assistants that provide contextually valuable information to help us complete tasks. Machine learning is the introverted superpower that has contributed to the significant progress of these chatbots and intelligent agents in recent years. While not commonly applied in learning environments, they are likely to become integral learning tools in time. Chatbot and virtual assistant platforms driven by ML and NLP techniques boast features that would be valuable in a learning context. Chatbots can be built and trained for learning without much technical development because other services provide much of the underlying platform. Chatbots also rely on much simpler user interface (UI) elements that do not require significant design and development. As they deliver large amounts of learning data, chatbots and intelligent agents offer extensive scope for additional integration of ML in learning.
There is no question that machine learning has the capacity to extensively enhance the HR function and, in particular, corporate learning and performance. When properly applied, ML can provide significant benefits across a wide range of learning and performance areas. It has the potential to provide more informed, reactive and effective learning platforms that can support the needs of today’s learners and drive a more data-centric approach to the delivery of learning, and learning strategy, within organisations.
While it is likely that an increased reliance on intelligent machines will make many current jobs redundant, their increasing integration into business workflows will create new jobs characterised by less manual effort – freeing humans to spend more time using their skills in critical thinking, analysis and creativity.
Talk to us
If you want to find out how Learnovate can help you apply machine learning to solve the challenges your company faces, talk to Tom at tom.pollock@learnovatecentre.org or +353 1 896 4910.